1University of Hohenheim · Institute of Animal Science, Master of Science
2Director Department of Animal Genetics and Breeding
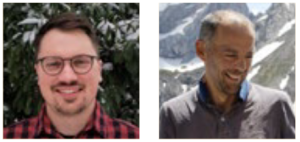
Valentin Haas studied Animal Science at the University of Hohenheim in Stuttgart, Germany. Here, he is currently a doctoral student at the Department of Animal Breeding and Genetics under the supervision of Prof. Dr. Jörn Bennewitz. His current research focuses on the relationships between host genetics, gut microbiota, and quantitative traits in poultry and other livestock species.
ABSTRACT
The influence of microorganisms in the gastrointestinal tracts of nonruminants has long been considered nonexistent or almost nonexistent. However, past studies have demonstrated the colonization of gut microbiota and its potential influence on efficiency traits in a wide variety of livestock species. Since efficiency traits are currently becoming more popular, the present study addresses the question of how such microbial information from nonruminants can be used in scientific approaches to animal genetics and breeding in the subject area of animal efficiency and performance.
The main current statistical methods and models that consider gut microbial colonization will be explained. Ways that quantify microbial influences on quantitative traits, that explain hologenomic (host genome plus microbiota genome) approaches and that consider causal relationships between microbial features along with efficiency traits and host genetics, are presented here.
INTRODUCTION
The efficiency of livestock production is of growing interest, especially in terms of resource conservation, environmental protection, animal welfare and food-feed competition. Feed efficiency can be divided into digestive and metabolic efficiency (Martin et al., 2021; Puillet et al., 2016). Digestive efficiency reflects the ability to absorb nutrients from ingested feed in the gastrointestinal tract (GIT) into the bloodstream, whereas metabolic efficiency is affected by allocation and reallocation processes, i.e., the conversion of absorbed nutrients into animal products.
The GITs of poultry harbor a variety of microorganisms (Apajalahti et al., 2004) influenced by external factors such as litter or diet (Borda-Molina et al., 2018; Kers et al., 2018) and by host genetics (Haas et al., 2022; Meng et al., 2014; Mignon-Grasteau et al., 2015; Wen et al., 2021; Zhao et al., 2013).
These members of the bacterial microbiota are not silent roommates, as they live in symbiosis with the host and are therefore involved in a number of processes in the digestive efficiency pathway, e.g., efficiency, utilization of nutrients, immune system and animal health (Maki et al., 2019; Rodehutscord et al., 2022; Stanley et al., 2014; Yadav and Jha, 2019). Figure 1. shows a summary of factors influencing the colonization of the GITs by microorganisms.
Factors influencing the animal gut microbiota.
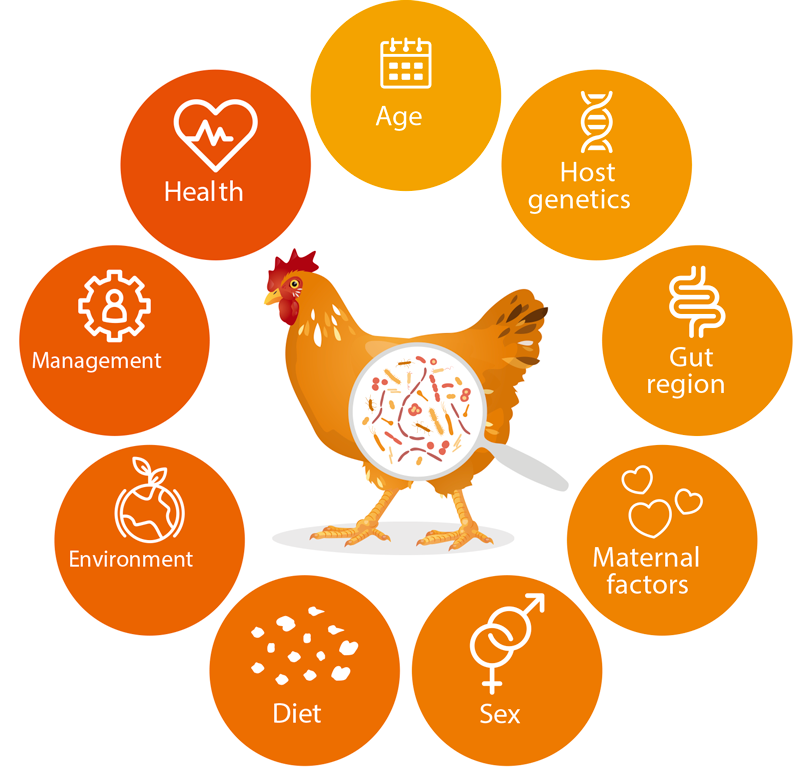
The consideration of microorganisms in the GITs of nonruminants can help improve the digestibility of various nutrients, as well as performance traits, and it seems to be beneficial to consider gastrointestinal microbiota in animal breeding (e.g., Haas et al., 2022; Khanal et al., 2020; Lu et al., 2018; Maltecca et al., 2019; Weishaar et al., 2020).
A deeper look at noteworthy statistical approaches in animal breeding that consider the bacterial colonization in the GITs of nonruminants (pigs and poultry) is compiled in this review. This review covers major microbial and genetic approaches but does not claim to be exhaustive.
MICROBIAL DATA
The interplay between the host, its gut microbiota and efficiency is complex and not yet fully understood. Nevertheless, the use of microbiota data has arrived in animal science and breeding. To use microbiota in genetic-statistical analyses, a powerful source of information is needed. In studies, mainly targeted amplicon sequencing of the 16S ribosomal RNA (rRNA) gene is applied (Borda-Molina et al., 2018; Maltecca et al., 2020).
For this, the DNA is extracted, and a specific target region (amplicons) of the small ribosomal subunit RNA gene is amplified. The 16S rRNA genes are conserved in microorganisms and contain a hypervariable region that allows for delineation of the microorganisms.
The resulting sequences are then clustered into operational taxonomic units (OTUs) based on bioinformatic processing using a similarity threshold, or the sequences are differentiated into amplicon sequence variants (ASVs) based on single nucleotide changes. Subsequently, the sequences for subordinate ranks (e.g., phylum, genus) can be taxonomically assigned. Therefore, deep characterization of microbiota communities and their quantification via relative abundances can be achieved.
Another common technique is metagenomic shotgun sequencing (whole-genome sequencing) (Borda-Molina et al., 2018; Maltecca et al., 2020; Pérez-Cobas et al., 2020).
This approach allows the parallel sequencing of DNA from all microorganisms in the ecosystem (GIT sample) with a high degree of coverage for species differentiation.
Metagenomics enables the collection of genomes and their corresponding genes and allows for the characterization of potential bacterial functions.
Since inexpensive and efficient sequencing methods to quantify the gut microbiota exist, the number of host microbiota studies has increased (Guevarra et al., 2019). The taxonomic classification of microbial data from the phylum to strain level is shown in Figure 2.
The GIT of poultry has various sections, with different digestion functions, and thus a differentiated settlement of microorganisms. The concentration of microbes increases in number from section to section and reaches its maximum in the paired ceca and the rectum (Yadav and Jha, 2019), and the distribution of the species in the different GIT sections is also different due to different habitat conditions (Yegani and Korver, 2008). For example, the crop, jejunum, and ileum are more likely to be home to bacteria of the family Lactobacillaceae and the caeca to the family Ruminocaccaceae (Witzig et al., 2015). Microbial data are given as compositional data (in relative abundances in percent), which means that the data per animal are multivariate and have a unit sum, i.e., an animal has 100 percent over all detected microbial features. Therefore, many animals in the microbiota dataset have a relative abundance of zero for some microbial characteristics, which complicates the use of microbial data for some analyses.
Taxonomic classification of microbial data from the phylum to strain level with the characterization potential
HERITABILITIES AND HOST GENETIC ARCHITECTURE OF MICROBIAL FEATURES
As stated above, the microbial colonization of the GITs of nonruminants is influenced by host genetics. Some studies have estimated significant narrow sense heritabilities for different GIT sections and different microbial features. Without any differentiation of the study environments and designs, the heritabilities differ at the GIT section and the microbial database used.
For example, heritabilities for bacterial genera in pig colon samples are in the range of 0.32 to 0.57 (Camarinha-Silva et al., 2017) and bacterial genera in fecal samples are in the range of 0.07 to 0.33 (Chen et al., 2018); in pig colons at the OTU level, the range is between 0.03 to 0.55 (Bergamaschi et al., 2020). Similar significant heritabilities could be found for chicken microbiota in fecal samples at the genus level, ranging from 0.21 to 0.79 (Meng et al., 2014), and Wen et al. (2021) reported different heritabilities in different sections of the GIT at the genus level: in the duodenum from 0.44 to 0.62, in the jejunum from 0.31 to 0.44, in the ileum from 0.38 to 0.79, in the cecum from 0.36 to 0.87, and in the feces from 0.41 to 0.71.
Lower heritabilities were reported in quail ileum at the genus level, from 0.04 to 0.17 (Haas et al., 2022). Additionally, heritabilities of the diversity (i.e., alpha diversity index) of the microbiota composition in pigs and poultry are shown in the literature in a range of 0.15 to 0.26, depending on the animal species, the respective GIT section, and the study design (Aliakbari et al., 2021; Déru et al., 2022a; Haas et al., 2023; Lu et al., 2018).
In addition to narrow-sense heritability, genetic correlation is also an important parameter in animal breeding. Several studies have estimated low to high genetic correlations between different microbial features and host efficiency traits. Aliakbari et al. (2021) and Déru et al. (2022a) found, for example, significant genetic correlations in pigs, Mignon-Grasteau et al. (2015) in chickens, and Haas et al. (2022) in Japanese quail.
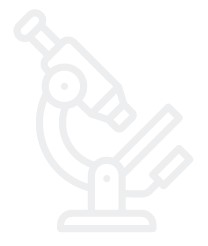
MICROBIAL RELATIONSHIP MATRIX M AND MICROBIABILITY
In animal breeding, heritability of the narrow sense is used to describe the relationship between the additive genotypic values of a trait and the phenotypic recording in a population. To quantify the relationship between the GIT microbiota and the recorded phenotype, the parameter microbiability was introduced by Difford et al. (2016).
Microbiability describes the part of the phenotypic variation of a trait that is explained by the microbial composition in the GIT. The approach to calculate microbiability, i.e., the proportion of microbial variance in the phenotypic variance, is equivalent to calculating heritability using the random animal effect in a linear mixed model. However, the random animal effect is modeled by a covariance structure from a microbial relationship matrix M rather than the pedigree-based/genomic relationship matrix used in the calculation of heritability. Different methods exist to build a microbial relationship matrix (He et al., 2022). A commonly used form is: M=1/NXXᵀ
with matrix X (n x N matrix, with n the number of animals and N number of microbial features), which contains the standardized and log-transformed abundances of the microbial features (Camarinha-Silva et al., 2017).
The animal microbiota correlation between two quantitative traits can be estimated with bivariate or multivariate microbial linear mixed models. Here, one does not use the pedigree-based/genomic relationship matrix as in genetic correlations but the microbial relationship matrix M. Medium to high animal microbiota correlations were found between different efficiency traits in quail (Vollmar et al., 2020) and pigs (Aliakbari et al., 2022; Déru et al., 2022b).Many studies have confirmed that the microbiome explains a substantial part of the phenotypic variation in efficiency traits (e.g., Verschuren et al., 2020 in pigs, Vollmar et al., 2020 in quail, and Wen et al., 2019 in chickens), and the animal microbial correlations are mostly significant and at high levels.
This enables the use of gut microbiota as a potential predictor of complex traits in animals.
MICROBIOME-WIDE ASSOCIATION ANALYSIS (MWAS)
Classical GWAS are used to detect trait-associated SNPs via mixed linear models. It is a common tool for the detection of genomic regions involved in the expression of quantitative traits (Schmid and Bennewitz, 2017). A method to detect trait-associated microbial features is microbiome-wide association studie (MWAS) (Tiezzi et al., 2021; Vollmar et al., 2020).
The relative abundance of a single microbial feature can be implemented as a fixed effect (fixed covariate) in a mixed linear model. Vollmar et al. (2020) modeled the random genetic animal effect in MWAS to model the population structure as in a GWAS.
The MWAS approach was designed to find parts of the respective microbiota class that are associated with the trait under consideration. The study of Vollmar et al. (2020)clearly showed that some substantial peaks could be found at the genus level. It should be noted that some microbial parts affected more than one efficiency trait, and some bacteria contributed more than others to the overall phenotypic variance of one trait. However, a polymicrobial influence is emerging in all traits.
This method can be used to identify trait-associated bacterial features. Aliakbari et al. (2022) confirmed the polymicrobial MWAS results for feed efficiency and performance traits in pigs, and Wang et al. (2022)found higher effects for some trait-associated genera on the fat composition of pigs.
It seems as if the identified microbial features are causal. However, the use of compositional data limits the identification of causality using MWAS results (Vollmar et al., 2020).
MICROBIAL TRAIT PREDICTIONS
When the microbiability of a quantitative trait is greater than zero, microbiome information can be used to predict the trait phenotypes by microbial best linear unbiased prediction (MBLUP) (Camarinha-Silva et al., 2017) or by machine learning approaches (e.g., Maltecca et al., 2019). This requires a large reference population comparable to genomic selection.
In most studies, MBLUP has similar or even higher prediction accuracy than comparable genomic BLUP for the same feed efficiency characteristics (e.g., Verschuren et al., 2020, Weishaar et al., 2020, Haas et al., 2022).
The main difference between microbial and genomic trait predictions is that the microbial predictions are not stable because the microbiota composition varies with the section of the GIT, animal age, and environmental factors (see Figure 1) (Maltecca et al., 2020; Weishaar et al., 2020).
Part of the microbial composition is determined by host genetics. Hence, there exists an overlap when selecting either microbial or genomic prediction values (Ross and Hayes, 2022).
HOLOGENOMIC APPROACH
Host genetics and the gastrointestinal microbiota can influence quantitative traits. Simultaneously, the microbiota composition in the GIT is influenced by host genetics, which means that there exists a relationship between these three features (Figure 3).
In most of the studies, these pathways were considered separately, either as genomic or microbial phenotypic predictions. However, we know that genetic and microbial variance are not independent, and therefore, to consider both direct genetic effects on a phenotype and indirect genetic effects via the microbiota, it may be beneficial to consider them together in a hologenomic approach.
Therefore, the hologenome represents the entirety of the DNA of the host (host genome) and the DNA of the gut microbiota (metagenome) (Bordenstein and Theis, 2015; Estellé, 2019).
The simplest conceivable method would be to put the genomic and microbial relationship matrices together in a mixed linear model, as was done, for example, in Aliakbari et al. (2022) and Déru et al. (2022b).
However, the problem is that interactions with independent covariates between markers and microbial features are not computable. Several studies have developed different methods to address this problem, and a brief overview is given in the following.
Weishaar et al. (2020) developed a two-step method that first used a microbial linear mixed model to predict the animal microbiota effect of the respective trait from microbial abundances and then a genomic linear mixed model to predict SNP effects for the previously predicted animal microbiota effect of the trait.
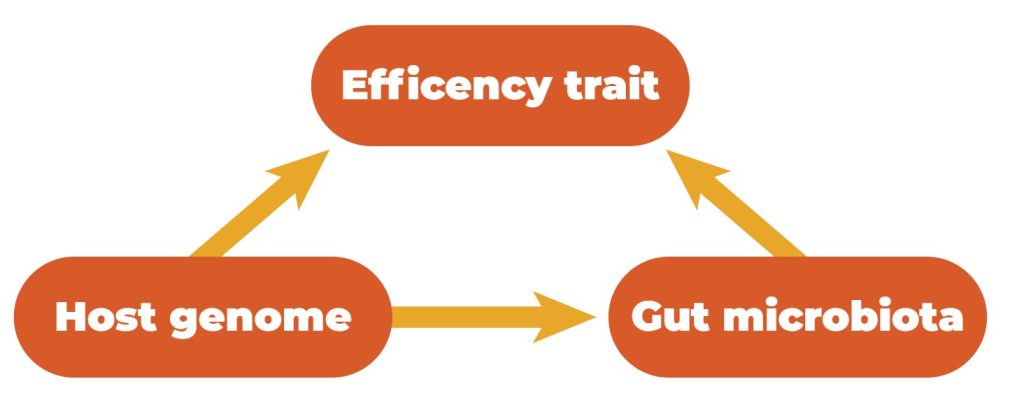
Relationships between efficiency traits, host genetics, and gut microbiota.
This method does not consider the genetic effects that directly affect the corresponding trait (not via the microbiome). For this reason, the authors used a selection index to separate the proportion of genes that influence the microbiota effects of a trait and the proportion of genes that directly influence the trait but not the microbes.
This addresses the genes that explain the trait not only directly through a change in metabolic pathways but also indirectly through a change in the composition of the gut microbiota.
This method was implemented to place more weight on the breeding values explained by the digestive pathway rather than the metabolic pathway, and selective breeding becomes possible.
A related method was developed by Christensen et al. (2021). In their study, the authors decomposed the breeding value of a quantitative trait into two genetic effects by directly estimating the microbiota-mediated breeding value and the residual breeding value of a trait, i.e., the genetic effects of the trait, without the genetic effects via the microbiome.
The difference between the two methods is that Christensen et al. (2021) estimated the residual breeding value in a linear mixed model, whereas Weishaar et al. (2020) did so in a final step via the selection index.
Khanal et al. (2020), Pérez-Enciso et al. (2021) and Qadri et al. (2022)considered both microbial features and host genetics together in one model and implemented different forms of interactions between host genomes and microbiomes.
The hologenomic selection approach mentioned above might enable the differentiated selection of the acquisition and reallocation process in animal efficiency. The goal is to adapt the trait-specific microbial colonization in the GIT to put more weight on the acquisition pathway for better utilization or usability from feed components that can then be used in the bloodstream.
This should somewhat reduce selection for metabolic efficiency, which creates a discrepancy between animal performance and health (Huber, 2018). However, metabolites produced by microorganisms can also alter the metabolic pathways of animals by producing short chain fatty acids.
Thus, microbiota can also affect the metabolic effect, not just the digestive effect of efficiency (Weishaar et al., 2020).
Hologenomic approaches considering only single traits and the interactions with other phenotypic expressions are of course not considered, and this still requires much research, but a first foundation to implement this in breeding practice has been developed.
In general, across the different studies, an improvement in the accuracy of trait prediction was observed when microbial data were included (e.g., Déru et al., 2022b; Khanal et al., 2020). However, there is still much room for improvement in the statistical representation of the covariance between the gastrointestinal microbiota and host genetics in terms of phenotypic traits.
The composition of the gut microbiota is partially under the genetic control of the host and can be used to improve genomic predictions of efficiency traits. Thus, it appears that the gut microbiota acts as a mediator between host genetics and efficiency traits, and it seems that the host genetic effect of efficiency traits is composed of a direct genetic effect of the trait and an indirect genetic effect via the modeling of the gut microbiota composition.
ARE GUT MICROBES THE CAUSAL DRIVER OF EFFICIENCY TRAITS?
A tool to differentiate direct and indirect effects between traits is structural equation models (SEM), as introduced in animal breeding by Gianola and Sorensen (2004). These authors used structural coefficients between trait combinations in multivariate mixed model equations to estimate the rate of change of trait i through the recursive influence of trait j, i.e., the rate of change of trait i by the change of one unit of trait j (Figure 4).
The combination of phenotypes, host genetics, and microbial data in structural equation concepts was done in most cases by using a linear mixed model approach, where the microbial features were considered as phenotypic trait records.
First, it was published by Saborío-Montero et al. (2020) in methane emissions in dairy cows and by Haas et al. (2022) in phosphorus utilization and related traits in Japanese quail as a model species.
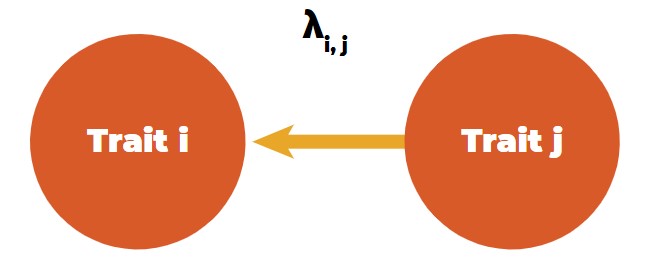
Recursive (directional) relationship between traits.
Low to high unidirectional relationships were found depending on the host species and traits under consideration.
Tiezzi et al. (2021) also confirmed direct and microbiome-mediated host genomic effects on backfat traits in swine by using GWAS and MWAS in a causal framework.
Looking at a pool of different correlated phenotypes, dependencies or causal relationships between the traits are not easy to identify, e.g., does the animal consume more food to perform as genetically predisposed or does the animal perform because of increased feed intake.
Bayesian networks can be used to detect such complex directional relationships between characteristics with a large dataset. Model residuals are used to remove any confounding variables such as gender, herd effect, test day effect, additive genetic effects, etc., that might distort the network (Rosa et al., 2011).
By using a Bayesian learning algorithm, we found a stable causal network for three efficiency traits (body weight gain, phosphorus, and calcium utilization), one bone ash trait (tibia ash), and two microbial phyla (Firmicutes and Actinobacteria) in an F2-cross of 750 Japanese quail (Figure 5).
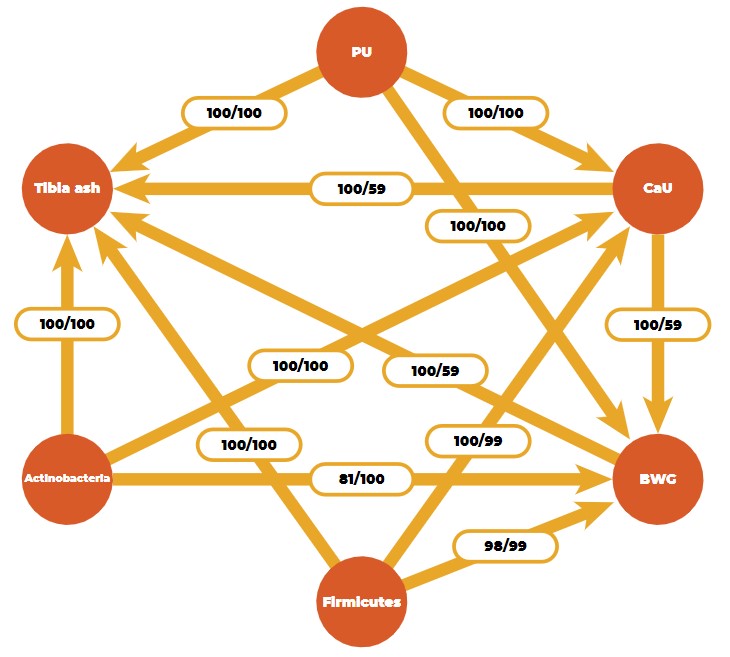
Causal network obtained from a Bayesian network learning algorithm (PU: phosphorus utilization, CaU: calcium utilization, BWG: body weight gain).
Numbers on the arrows are bootstrap sample proportions (50,000 samples) indicating an existing arc (figures on the left) and the proportion of the direction (figures on the right). The causal structure between the efficiency and ash traits is logical from a biological point of view, but the connection with microbial features is novel.
Such causal networks can then be used in conjunction with SEM to quantify unidirectional relationships, which shows how host genomes can be used to map and understand causal structures among quantitative and microbiota traits.
These results of the SEM can then be extended to SEM-GWAS on the basis of the causal network in connection to a GWAS, where the differentiation of SNP effects into direct and indirect effects can be performed (Momen et al., 2019; Pegolo et al., 2020).
Many indirect SNP effects, which directly influence an upstream trait and exert an indirect influence via the recursive relationship, are probably not detected by a classical GWAS (Tiezzi et al., 2021).
OUTLOOK: FURTHER TRAIT COMPLEXES FOR HOLOGENOMIC BREEDING
The studies undertaken thus far in poultry and pigs have mainly considered feed or nutrient efficiency-related traits.
Considering the effects of the microbiota on animal health, microbial colonization in the gut is closely linked to the immune system, e.g., the energy supply of the immune system through the production of short-chain fatty acids and the anti-infection barrier by inhibiting pathogens (Diaz Carrasco et al., 2019; Shang et al., 2018).
Future breeding strategies for improved immune systems could consider microbial colonization in the GIT and stabilize a natural barrier to unwanted microorganisms, especially in the gut habitat (Yegani and Korver, 2008).
For example, in poultry production, Salmonella and Campylobacter are unwanted microorganisms with zoonotic potential and some antibiotic resistance (Shang et al., 2018).
Other studies have considered the GIT microbiota in terms of complex behavioral characteristics such as feather pecking or aggressive pecking (e.g., van der Eijk et al., 2020, Borda-Molina et al., 2021, Mindus et al., 2021). The authors argued that certain microorganisms in the GITs of animals can influence behavior or stress response via hormonal changes through the gut-brain axis.
A broader consideration of social or hormonally influenced behaviors in relation to microbiota composition may become more important in the future.
However, such concepts still need substantial research. Further development or increased research in the field of hologenomic selection for metabolic and digestive efficiency could possibly also enable a better understanding of the current welfare debate in poultry breeding.
An example could be keel bone fractures in laying hens, since phosphorus utilization is influenced by the gut microbiota (Haas et al., 2022; Vollmar et al., 2020) and tibia and foot ash have significant microbiabilities (not yet published).
The collection of gastrointestinal microbiota data for trait prediction is not easy to capture, and for some phenotypic observations, the useability of simple fecal samples is restricted. The main absorption in the GIT takes place in the small intestine. However, microbiota sampling in this section on live animals is invasive or can only be cultured from dead animals, which is why fecal samples are used in many studies (e.g., Déru et al., 2022b). A potential way would be the prediction of microbial information of the desired gut section via microbiota from noninvasive sampling, e.g., cloaca samples. Andreani et al. (2020) found, for example, that cloaca samples are good for predicting cecal microbiota in chickens, which needs further investigation.
Another solution would be to collect only standardized DNA samples from selection candidates and, together with a large reference population with known microbiota, host DNA, and phenotypic information, predict the phenotypes of the selection candidate.
This restricts one to what is possible from a breeding point of view. A way to do so is the hologenomic selection approach discussed above. In the future, technical sampling solutions such as osmotic pills, which are inserted into the GIT by mouth and can take samples in the desired section using an external magnet, may play a role (Rezaei Nejad et al., 2019).
CONCLUSIONS
Part of the gastrointestinal microbiota is influenced by host genetics, which allows for breeding. The microbial composition in turn influences some quantitative traits, and the microbiota thus acts as a mediator between host genetics and quantitative traits.
The consideration of the microbial composition could therefore be worthwhile in breeding approaches.
Challenges include the expensive and laborious microbial phenotyping of high numbers of animals and the lack of understanding of the relationship between complex quantitative traits and the GIT microbiota.
订阅我们的新闻稿
了解所有最新行业新闻。